Sravana Reddy
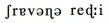
I am a researcher with interests in natural language processing and machine learning. I currently work at Spotify as a research scientist. I've also worked at a startup, ASAPP, and in academia. I graduated with my PhD from The University of Chicago.